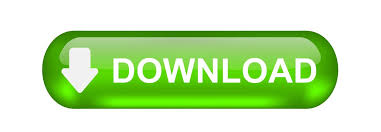
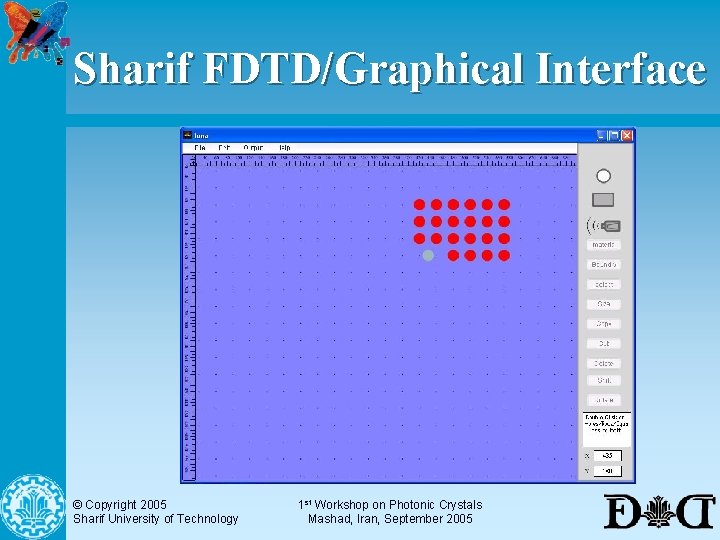
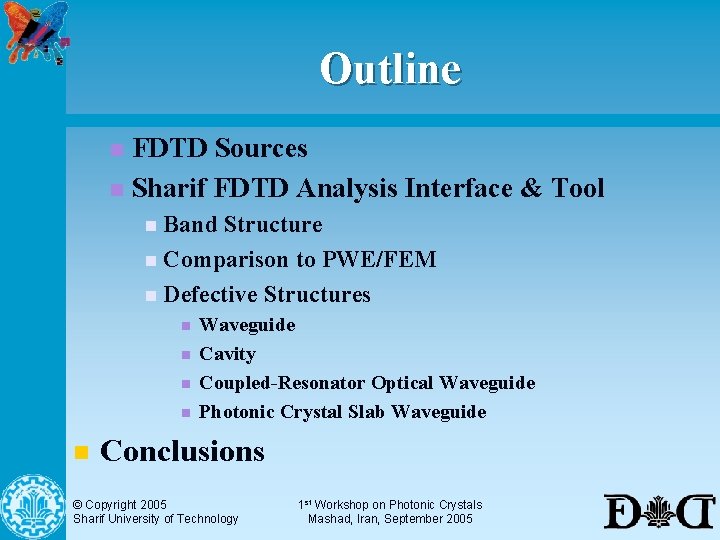
Phototoxicity is strongly related to molecular photochemistry and photostability 2. The model was trained with the TD-DFT results of about half a million molecules. 6 used random forests to predict the highest oscillator strength and associated excitation energy among ten excitation states of molecules from 1 and 2D descriptors of the molecule. 5 calculated a database of 132 k excitation spectra using the PBE density functional augmented with vdW corrections, and trained neural networks with various architectures to predict the spectra from the 3D structure. 4 The models were explored both to predict structural parameters for a given spectrum and to predict a spectrum for given structural parameters. ML models based on theoretical optical spectra pre-calculated by finite-difference time-domain (FDTD) simulations for gold nanoparticles and nanorods were reported by Pashkov et al. ML algorithms can also be trained with theoretically calculated data sets obtained, e.g., by DFT methods, for faster estimation of molecular properties 8, 9, 10, 11. Another database of experimental and computational UV–Vis absorption spectra attributes was recently obtained through mining methods 7. A comparison between predictions of the absorption and emission spectra of coumarin 153 in ethanol using the ML model and TD-DFT calculations revealed a better performance of the ML model when compared to the theoretical calculations 3. The root mean squared errors of the predicted values were found to be 26.6 and 28.0 nm for absorption and emission peak positions. 3 reported a deep learning model developed with an experimental database of 30 ,094 chromophore/solvent combinations to predict several optical properties, namely, the first absorption peak position, bandwidth, and extinction coefficient, the emission peak position, bandwidth, and photoluminescence quantum yield and illustrated the possibilities of applying ML to find target molecules with desired optical and photophysical properties.

Machine learning (ML) techniques have been reported for the prediction of optical and photophysical properties of organic compounds 3, 4, 5, 6. The prediction of UV–Vis spectra from the molecular structural formula is of general high interest to design new materials, identify potential phototoxic compounds, estimate missing spectroscopic data for known molecules, or curate databases of experimental spectra.
#Fdtd analysis skin#
In the human body, the combined effect of an external chemical compound (e.g., plant toxins, phytomedicines, cosmetics, agrochemicals, food additives, dyes, drugs) and exposure to light, especially ultraviolet and visible radiation may give rise to an acute unwanted response in the skin or retina, which is called chemical phototoxicity 1, 2. The UV–Vis absorption spectrum is a key physical property of an organic compound that determines many of its optoelectronic properties and photochemical reactivity. Comparable results were observed with the classification directly based on experimental UV–Vis data in the same format. The ML output (UV–Vis spectrum class) was explored as a predictor of the 3T3 NRU phototoxicity in vitro assay for a set of 43 molecules. The models were validated with two external test sets comprising 998 organic molecules, obtaining a global accuracy up to 0.89, sensitivity of 0.90 and specificity of 0.88. Random forests were selected among several algorithms. They were labeled with positive class (related to photoreactive potential) if an absorption maximum is reported in the range between 290 and 700 nm (UV/Vis) with molar extinction coefficient (MEC) above 1000 Lmol −1 cm −1, and as negative if no such a peak is in the list.
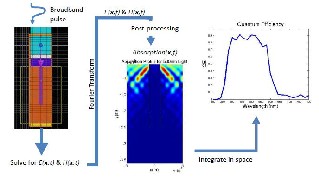
Training and test data (~ 75 k molecules and associated UV–Vis data) were assembled from a database with lists of experimental absorption maxima. Machine learning (ML) algorithms were explored for the classification of the UV–Vis absorption spectrum of organic molecules based on molecular descriptors and fingerprints generated from 2D chemical structures.
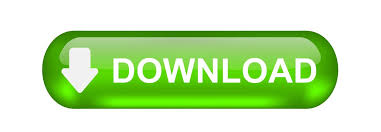